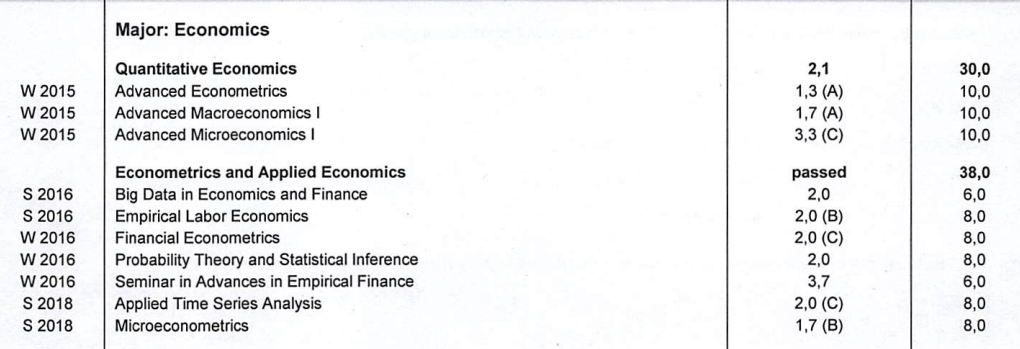
Nowadays financial institutions use data at the transaction level for algorithmic trading. In order to predict the movements of stock market appropriate short-run (“as long as the breath is warm”) forecasting methods are applied. One of the challenging issues is to account for many predictors and the discreteness of events and prices. Multi-response models can be implemented to fit this type of models. As the high correlated structure remains steady through covariates, naïve way of estimating parameters and creating possible forecasting tool will mostly be a failure (non-existent MLE functions, infinite variances, ill-conditioned matrices). Shrinkage methods (Ridge, Lasso, etc.) are one of the best ways to deal with ill-conditioned situations. They can perform well even when number of parameters are larger than the number of observations (p > N). In this research, I investigate the predictive power of wide range of models in penalized multinomial framework. Existing literature on direction of change are mainly using two categories for responses, up and down movements. As opposed, I implement as many categories as possible to account for any possible predicted change, each category defined as an interval of possible outcomes (for price data, each return interval represents a category, the more the category the smaller the interval). I had a preliminary research on high frequency stock price, implementing as many as 9 categories due to speed limits and size of the data (around 300,000 observations). Over a range of models (with different categories at most being 9) from LASSO to RIDGE (and special case of ridge with symmetric side constraints), examining predictive power on out-of sample gives an idea on how powerful regularization can be in terms of prediction. I should emphasize the fact that in these studies, meaning of parameters and the models are not discussed. I am currently extending my research using simulation methods for different scenarios. One of the main interests of mine is questioning ‘what if there are many more categories?’. By ‘many more’, I do not want to limit the imagination, yet I am currently working on 50 to 100 categories (from simulated data). This might give a better picture, since it gives a distribution-like measure for each prediction. As many categories, as we utilize, each predicted probability for each category, that is interval, serves as a possible outcome of a set of covariates. This kind of usage can be extended to other possible fields as well. One main problem would be computational burden for sure with real highly frequent data. The data does not have to be highly frequent for applications of this kind. For any event, future possible outcomes can be categorized and estimated this way (yet theoretically we can build thousands of categories with high frequency data). Further research (I am working on it simultaneously, as it is related) is needed for analyzing high frequency data in terms of scaling-law approaches as well. As we go subatomic level in data, more and more frequent data can yield different scaling laws that might give an insight more about what we need to explore about the highly frequent data.
References
Friedman, J., Hastie, T., & Tibshirani, R. (2010). Regularization Paths for Generalized
Linear Models via Coordinate Descent. Journal Of Statistical Software, 33(1).
http://dx.doi.org/10.18637/jss.v033.i01
Glattfelder, J., Dupuis, A., & Olsen, R. (2011). Patterns in high-frequency FX data:
discovery of 12 empirical scaling laws. Quantitative Finance, 11(4), 599-614.
http://dx.doi.org/10.1080/14697688.2010.481632
Zahid, F. & Tutz, G. (2012). Ridge estimation for multinomial logit models with
symmetric side constraints. Computational Statistics, 28(3), 1017-1034.
http://dx.doi.org/10.1007/s00180-012-0341-1
Bisig, T., Dupuis, A., Impagliazzo, V., & Olsen, R. B. (2012). The scale of market
quakes. Quantitative Finance, 12(4), 501-508.
Aloud, M., Tsang, E., Olsen, R. B., & Dupuis, A. (2011). A directional-change events
approach for studying financial time series. EconomicsDiscussion Paper, (2011-28)